As investments in AI use cases in financial services continue to pick up the pace, generative AI is to remain the center of executives’ attention in 2024. The technology is powering new forms of self-service customer support, robo-advisors and virtual assistants, and productivity tools.
AI in general continues to be an efficiency booster across financial services organizations. Its use in fraud detection, underwriting, biometrics, and customer analytics remain among the top applications of AI in finance in 2024.
The proliferation of AI in finance also attracts the attention of regulators. In addition to general AI regulations (e.g., the EU’s AI Act), financial watchdogs around the world issue guidelines on AI use in finance.
Consumers in developed markets, however, remain unconvinced by AI. Countries like the United States, France, Australia, and Canada score low on excitement over AI and high on concern with the technology
The two issues are the main force behind the push for ethical AI use in finance. It stands for implementing explainable, fair, robust, and transparent models that protect privacy and ensure data security. Financial services, however, also have to deal with data issues, talent shortages, and budget constraints to seize the opportunities of AI.
About S-PRO
We are a software development and IT consulting partner for businesses in financial services, healthcare, and renewable energy. We leverage over a decade of full-cycle development experience to help our clients leverage AI, blockchain, big data, cloud, and other cutting-edge technologies.
We enable fast-growing startups and enterprises to scale operations, boost efficiency, transform customer experiences, and launch innovative products.
Learn more about our work from our case studies.
Recent AI Developments to Drive Innovation in 2024
2023 marked the continuous development of generative AI, along with the emergence of causal and constitutional AI.
Generative AI
According to Gartner’s 2023 AI Hype Cycle, generative AI continues to dominate the AI discussion but has reached the peak of inflated expectations. However, that doesn’t mean it’s time to discount it altogether. Generative AI in finance and accounting can power:
- Faster digital transformation with code writing assistants (e.g., GitHub Copilot)
- Synthetic data generation for model training, anomaly detection, and cybersecurity
- Report generation and summarization for better customer onboarding
- Personal virtual assistants that cater to customers’ daily needs 24/7
Generative AI continues to develop at a rapid pace. The most recent innovations in this domain include:
- Multimodal AI models that can generate output based on multiple types of data as input (e.g., OpenAI’s GPT-4V can switch between NLP and computer vision tasks)
- Text-to-video generative AI models (e.g., Google’s Lumiere announced in late January 2024, Runway’s Gen-2 model)
- Smaller language models that are less resource-intensive yet deliver better performance when trained on large data sets.
Causal AI
Unlike other forms of artificial intelligence used to predict behavior and outcomes, causal AI aims to find the root causes of documented outcomes. In other words, while predictive analytics models usually look for correlation, causal AI is trained to identify cause-and-effect relationships. This allows it to predict future outcomes with more accuracy and autonomy.
Furthermore, causal AI is, by default, explainable. A human supervising the model can trace its decision-making process and understand why the algorithm produced a given result. This resolves a common issue AI researchers and developers struggle with – the black-box problem.
Causal AI can tackle a variety of tasks in the finance industry, such as:
- Identifying customer churn causes
- Improving the accuracy of risk assessment in loan underwriting
- Enhancing the accuracy of fraud detection
Constitutional AI
Avoiding algorithmic bias and inaccurate results typically requires a tremendous amount of human feedback in the reinforcement learning framework with human feedback (RLHF). Using human judgment, it allows for weeding out harmful, inaccurate, or unhelpful results.
However, this method isn’t infinitely scalable. On top of that, if human supervisors can’t pinpoint how the algorithm arrived at its conclusion, the efficiency of human feedback drops.
Constitutional AI seeks to align the AI models with human values more efficiently while addressing these two issues. It involves outlining the values the AI model has to abide by and then training it to rate the output based on the alignment with those values. The model is then incentivized to produce results that score higher in this alignment.
Constitutional AI can be a response to the demand for ethical and responsible AI. For example, Claude, a chatbot developed by Anthropic, was aligned using the constitutional AI methodology.
Regulatory Landscape Is Evolving
AI use in finance has never been completely unregulated. Financial institutions have to abide by the same data privacy laws and industry-specific regulations when deploying AI as in any other undertaking.
That said, 2024 is bound to be the year of increased attention to AI applications in finance on regulators’ part – and mounting regulatory pressure.
Emerging AI Deployment Regulations
AI has managed to capture legislators’ attention across the globe. The mentions of the technology in legislative proceedings almost doubled between 2022 and 2023.
The European Union is paving the way with its recently adopted Artificial Intelligence Act (AIA). Brazil, Canada, China, and South Korea also have general AI legislation in the works.
EU’s Artificial Intelligence Act Is In
The European Union’s Artificial Intelligence Act (AI Act) is, arguably, the most ambitious regulatory framework for AI systems so far. Adopted by the European Parliament in March 2024, the AI Act introduces the notion of high-risk AI systems.
Implementing such systems has to involve:
- Proper risk assessment and mitigation
- Continuous log maintenance
- Algorithm transparency and accuracy
- Human oversight
For instance, the AI Act lists AI systems for assessing creditworthiness in banking or evaluating risk and calculating prices in insurance underwriting as high-risk systems. Their developers are then obligated to:
- Implement data governance and ensure datasets are representative, error-free, and complete
- Establish a risk management system
- Allow for human oversight in their systems
- Ensure high levels of system accuracy, security, and robustness
- Implement a quality management system
U.S. Is Ramping Up Regulatory Pressure
President Biden’s recent executive order sets the tone for further AI regulation in the United States. The executive order directed federal agencies, including the U.S. Treasury, to review and draft new rules for AI use across multiple industries.
However, any federal legislation on AI use is unlikely to pass during the election year, according to Deloitte’s 2024 capital markets regulatory outlook.
That said, U.S. industry regulators are equally unlikely to leave organizations to their own devices when it comes to the use of AI in finance:
- Deloitte expects the U.S. Consumer Financial Protection Bureau (CFPB) to scrutinize the use of AI in loan decision-making in 2024.
- The National Association of Insurance Commissioners (NAIC) adopted the Model Bulletin on the Use of AI Systems in late 2023. The document serves as a template for state insurance regulators to pass their own laws.
- U.S. Treasury published a report on AI-related risks, challenges in cybersecurity and fraud, and the best practices for addressing them in March 2024.
OECD Principles Remain a Good Benchmark
On the global scale, the AI principles and recommendations adopted by the OECD and G20 in 2019 continue to serve as a benchmark for regulators across jurisdictions. These principles include:
- Respect for human rights, democratic values, and diversity
- Inclusion of safeguards, such as enabling human intervention
- Transparency and responsible disclosure around AI systems
- Continuous risk assessment and management
Figure 1: Summary of OECD Al principles and recommendations to governments
OECD Al principles | OECD recommendations to governments |
---|---|
Al should benefit people and the planet by driving inclusive growth, sustainable development, and well-being. | Facilitate public and private investment in research and development to spur innovation in trustworthy Al. |
Al systems should be designed in a way that respects the rule of law, human rights, democratic values, and diversity, and they should include appropriate safeguards - for example, enabling human intervention where necessary - to ensure a fair and just society. | Foster accessible Al ecosystems with digital infrastructure and technologies, and mechanisms to share data and knowledge. |
There should be transparency and responsible disclosure around Al systems to ensure that people understand when they are engaging with them and can challenge outcomes. | Create a policy environment that will open the way to deployment of trustworthy Al systems. |
Al systems must function in a robust, secure, and safe way throughout their lifetimes, and potential risks should be continually assessed and managed. | Equip people with the skills for Al and support workers to ensure a fair transition. |
Organizations and individuals developing, deploying or operating Al systems should be held accountable for their proper functioning in line with the above principles. | Co-operate across borders and sectors to share information, develop standards and work towards responsible stewardship of Al. |
Existing Regulations Setting the Framework
Data privacy regulations continue to set the boundaries for AI use across jurisdictions:
- In the U.S., the California Consumer Privacy Act (CCPA) enables California residents to opt out of the use of their personal information. Colorado and Virginia state privacy laws give their residents the same rights. Connecticut’s legislation allows residents to opt out of fully automated decision-making.
- In the EU, the General Data Protection Regulation (GDPR) introduced the obligation to handle data securely and the right to be forgotten. It also requires organizations to obtain consent for personal data processing.
In addition to that, organizations have to be mindful of other legal requirements that may apply to their AI use, such as:
- Anti-money laundering (AML) regulations
- Know Your Customer (KYC) requirements
- Consumer protections (e.g., FAIR Plans requirements in certain U.S. states)
IT security requirements for financial institutions are also in the crosshairs of some regulators. In the European Union, for example, financial instructions will have to comply with the Digital Operational Resilience Act (DORA) by January 17, 2025.
DORA covers requirements toward:
- ICT risk management, including third-party risk management
- Digital operational resilience testing
- Incident handling
- Information sharing
How to Use AI in Finance: 10 Use Cases
How is AI used in finance? Here are the ten AI applications in finance to keep an eye on in 2024, along with real-world examples of organizations leveraging the benefits of AI.
Self-Service Customer Support
Customer support can be expensive. AI-powered customer support chatbots, however, save $8 billion in costs annually for financial services organizations, or $0.70 per interaction.
Chatbots can reduce the volume of simple requests handled by human customer support agents. As a result, they can focus on the complex requests and issues that chatbots can’t handle all that well.
That said, chatbots aren’t a panacea. They are also the number one source of friction in digital experiences in the industry, according to Salesforce.
AI-powered chatbots leverage conversational AI and natural language processing to deliver responses to customers’ queries. Large language models (LLMs) are here to power a new generation of customer support chatbots, too – ones that can respond like a human would, 24/7.
In addition to text-based chatbots, voice recognition technology can power the first line of contact in phone support. As another application of AI in the finance industry, such voice assistants can respond to common questions, reducing the strain on call centers, and reroute only complex queries to human support agents.
Examples
- BNP Paribas Cardif’s Cardi bot was designed to assist customers who contact the insurer in the case of loss events. The bot is a voice assistant that responds to common queries, such as how to get a payout. The bot reportedly saved BNP Paribas Cardif over $9 million in operational costs over two years.
- Capital One’s chatbot assistant, Eno, helps customers protect their accounts by alerting them about suspicious activity. It can also ask for a confirmation to proceed with a transaction – something traditionally done by call center agents. Finally, the chatbot can lock and unlock the credit card per user’s request.
Robo-Advisors/Virtual Assistants
Robo-advisors and virtual assistants are designed to give customers personalized advice on anything from investment choices to personal finance management. They’re made possible with a combination of conversational AI, natural language processing, and machine learning.
While generative AI makes the robo-advisors’ responses even more lifelike, it’s not the only AI technology required to make it work. This application of AI in finance also needs machine learning and predictive analytics to identify the most suitable advice based on the initial parameters.
For example, a robo-advisor may be designed to pinpoint the most suitable investment options for a particular customer. In this case, the system has to assess their investment goals and risk tolerance, along with the market conjuncture, to make a recommendation.
Examples
- JPMorgan is developing a GenAI chatbot that would help its customers select investments. Dubbed IndexGPT, the chatbot will analyze and select securities based on customer needs, essentially automating the role of a financial advisor.
- Wells Fargo launched its Fargo virtual assistant in March 2023, and it hit 20 million interactions by January 2024. Powered by GenAI, it can execute tasks like paying bills, providing transaction details, and answering everyday banking questions.
- Magnifi is an AI-powered personal investment aggregator that offers AI portfolio analysis and a conversational AI virtual assistant to its users.
- Wells Fargo also has another LLM-powered chatbot called LiveSync. It serves as a goal-setting and financial planning assistant and is an example of using AI in personal finance management.
- Bank of America’s virtual financial assistant, Erica, handles 2 million interactions a day. Launched in 2018, it notifies customers about credit score changes, allows them to view and redeem rewards, and provides weekly updates on monthly spending, among other things.
Productivity Tools
In investment banking alone, generative AI is poised to increase front-office employees’ productivity by 27-35% by 2026, according to Deloitte’s research. Another study, conducted by Stanford University, revealed that customer support agents working with an AI assistant resolved 13.8 more issues per hour.
Customer support agents aren’t the only frontline workers poised to become more productive with the help of generative AI applications. Marketers, data analysts, and consultants can all benefit from GenAI, both in terms of reducing time and improving the quality of results.
Furthermore, in-house developers can leverage GenAI copilots to streamline code writing and debugging.
Examples
- Goldman Sachs is experimenting with GenAI to boost its developers’ productivity. The tool is meant to help developers automatically generate and test code, with the share of AI-generated code reaching as high as 40% in some cases. Goldman Sachs’ CIO affirmed in 2023 that the company’s developers are already using this assisted coding technology.
- AXA rolled out SecureGPT, which is based on Microsoft’s Azure OpenAI Service, to its employees in June 2023. SecureGPT enables users to sum up, translate, refine, and generate texts within seconds.
Fraud Detection
According to Nasdaq’s Global Financial Crime Report, fraud resulted in $485.6 billion in losses globally in 2023 alone. AI in banking and finance has long been used to power fraud detection and prevention through machine learning-powered data analytics.
AI algorithms can detect potential fraud in real time and immediately trigger a review. They also spot suspicious activity with a higher accuracy rate than a human could on a scale too large for a fraud detection team to handle manually.
As a result, AI brings down fraud detection costs, reduces manual auditing needs, and improves fraud detection rates.
That said, a human in the loop is still necessary to ensure false positives don’t harm customer experience and increase compliance risks.
Examples
- PayPal has been using AI in fraud detection and prevention for over a decade – in the form of deep learning models. From 2019 to 2022, its deep learning models halved the company’s loss rate, even as its annual payment volumes almost doubled in the same period.
- American Express uses GPU-accelerated long short-term memory (LSTM) models trained on NVIDIA DGX and optimized with TensorRT to identify potential fraud. This allows the bank to detect suspicious transactions in real time and with an accuracy higher by up to 6%.
Streamlined Underwriting
Much like AI models can assess the likelihood of a transaction being fraudulent, they can also analyze the potential risks for underwriting purposes – all in the blink of an eye.
To that end, machine learning models assess financial and other data to determine associated risks for every customer. Simultaneously, natural language processing (NLP) and optical character recognition (OCR) allow for the automatic extraction of data from scanned documents and unstructured data formats.
In insurance, AI-powered underwriting can reduce friction as the customer can get insured almost instantly thanks to fully automated price calculations. Furthermore, the variety of data points and parameters allows for fine-tuning pricing to every policyholder’s risk profile.
Banks and credit unions can also leverage this AI application in finance to assess risk and creditworthiness for loans and credit cards, even if the borrowers are non-prime and unbanked.
Examples
- Lemonade, an insurtech startup, claims that its underwriting algorithm collects and processes 100x more data points than traditional insurers. Its chatbot can complete the underwriting process without any human involvement, insuring customers within seconds.
- Rocket Mortgage, a Detroit-based mortgage lender, uses generative AI, automation, and machine learning to collect and process information for mortgage underwriting. The AI system, dubbed Rocket Logic, saves its underwriters 5,000 hours a month.
Physical & Behavioral Biometrics
Synthetic identity theft is the fastest-growing type of financial crime in the United States, with expected losses from it projected to reach $23 billion by 2030. In 85% of cases, synthetic identities bypass detection by third-party fraud models used in the consumer banking sector.
Biometric data now emerges as a way to combat synthetic identity theft and ramp up fraud detection and prevention. As a relatively new use case for AI in the finance industry, biometric verification can rely on iris, fingerprint, facial, or voice recognition to confirm the identity of the customer.
Biometrics can streamline customer onboarding, reauthentication, transaction authorization, and ATM operations, to name a few.
However, banks have to step up their game to avoid creative workarounds, such as bypassing FaceID’s attention detection with fake eyes. One way to do it is through behavioral biometrics, which tracks the user’s touchscreen behavior, navigation, and typing habits. With the help of AI-powered analytics, behavioral biometrics tools can spot discrepancies in user behavior.
Examples
- JPMorgan launched a pilot program for biometrics-based checkout in retail in March 2023. The program would enable customers to pay for their purchases using palm and facial recognition in select U.S. stores.
- Mastercard launched a Mastercard Biometric Card that combines chip technology with fingerprint recognition. The card contains a sensor that recognizes the cardholder’s fingerprint during in-store purchase authorization.
- BNP Paribas also began issuing biometric payment cards in all of its branches in 2021. Biometric authentication allows for contactless payments on purchases that exceed the 50-euro threshold.
Usage-Based Insurance
The combination of Internet-of-Things sensors, AI-powered real-time analytics, and advanced risk assessment models make for one of the most disruptive AI use cases in finance – in its insurance sector, to be specific. This combination gives rise to a new type of insurance product: usage-based insurance (UBI).
Usage-based car insurance involves software systems that collect and analyze telematics data from vehicle sensors or the policyholder’s smartphone. That data includes:
- Speed
- Acceleration
- Hard braking and cornering
- Distance driven
- Time of day
- Phone use
The data assesses how much the policyholder drives and how risky their driving behavior is. The company updates the pricing regularly (e.g., once a month) to reward safer drivers with lower premiums.
Examples
- Tesla entered the finance industry with its real-time insurance offering in the United States (except for California). It uses driving behavior to recalculate policyholders’ premiums every month. The Safety Score quantifies how safely the customer drives and takes into account the distance and vehicle driven and the driver’s location.
- Geico’s EasyDrive allows customers to purchase UBI regardless of the type of vehicle they drive. To that end, the insurer requires policyholders to install a smartphone app that calculates a driving score. The score is based on driving behavior metrics like hard braking, distracted driving, time of day, smoothness, and route consistency.
360-Degree Customer View
Gaining a better understanding of customers is another use case for AI in the finance industry – one that leads to reduced churn rates, increased customer lifetime value (CLV), and improved net promoter scores.
AI can help make sense of swaths of customer data to:
- Predict the likelihood of churn
- Identify the most appropriate products and services to recommend to the customer based on the customer journey stage, interactions, preferences, and financial goals
- Sort customers into micro-segments to improve the efficiency of marketing messages
- Analyze customer data across sources to gain a full-picture view of their needs and expectations
All this data, along with AI-powered analytics, allows for better customer experience personalization. And financial services consumers are craving it, according to Salesforce’s recent survey.
Examples
- Personetics, a fintech startup, leverages AI/ML and accelerated computing to analyze transaction data available under open banking regulations in real time. The platform serves as an aggregator of data from multiple API endpoints, categorizing it and sharing it with banks to enable them to provide more relevant recommendations.
- Capital One leveraged AI to resolve the conversion attribution problem in automated personalized online advertising. Its recommendation engine has demonstrated a better efficiency rate in targeting repeat visitors to the bank’s homepage.
Trade Operations Automation
AI in the finance industry – in the form of OCR, ML, and real-time data analytics – can automate trade reconciliations and exception management. This facilitates the move to the T+1 trade settlement cycle for financial institutions, including corporate finance players, as per regulatory requirements.
With the help of AI in corporate finance, AI tools can analyze transaction data aggregated from multiple sources to automate reconciliation. On top of that, OCR can extract data from scanned documents, eliminating the need for manual data entry.
AI-powered tools automate the process, reduce costs, and accelerate the trade settlement cycle. They also reduce the risk of human error.
One AI in finance research paper found predictive analytics to be a common tool in forecasting stock prices, performance, and volatility, as well. Such AI systems can provide investment managers with timely insights. Combined with automation, real-time analytics systems can automate buy and sell when certain conditions are met in what’s known as algorithmic trading.
Examples
- SmartStream, a long-time post-trade software vendor, implemented AI reconciliations in SmartStream Air. The system leverages AI to auto-configure reconciliations, extract data from multiple sources via API endpoints, and return a list of unmatched records within seconds.
- Capitalise.ai is a text-based trading analysis and automation tool that allows setting up algorithmic trading rules using everyday English. It comes packed with research and analytics tools that derive insights from real-time market data.
Multimedia Claims Processing
AI-powered computer vision powers the evolution of claim processing. Instead of sending an adjuster to assess the damage in person, the company can request the policyholder to send photos and videos of the insured property. Those can then be processed by an AI solution that returns a repair estimate in a matter of seconds.
Apart from assessing damage, this application of AI can be leveraged to extract additional data that can be instrumental in claims processing. For instance, a computer vision system can automatically detect the environment (highway/parking lot/etc.) and weather conditions from dashcam footage.
Computer vision systems can also process drone footage to streamline property damage assessment. Besides providing evidence for a more objective estimate, drone use reduces risks for the insurance company adjusters and expedites the process.
Examples
- Tractable is an insurtech startup that offers a visual damage assessment tool to insurers. One Tractable’s solution can compare and model property damage based on images and footage provided by customers or captured using drones. Another one can identify which car parts can be salvaged after an accident.
- CCC trained a custom deep learning model on its billions of photos of damaged vehicles, along with claim form data and incident reports. The model delivers repair cost recommendations based on the patterns identified in the historical data.
How the AI in Finance Market Is Doing in 2024
The global market size of AI in fintech reached $42.83 billion in 2023 and grew to $44.08 billion, according to one estimate. The banking and financial services industry is the second-largest segment of the global AI market, which was valued at $196.6 billion in 2023.
The AI in finance market is expected to grow to over $50 billion by 2029, representing a 2.91% compound annual growth rate (CAGR). However, its generative AI segment is to outpace that growth.
GenAI in the finance market was valued at only 0.85 billion in 2022. However, it’s projected to reach over 9.4 billion by 2032, representing a 28.1% CAGR.
The State of AI Adoption in Finance
48% of financial services organizations already use AI in operations, and 45% have adopted the technology in risk and compliance. The runners-up are marketing (34%) and sales (27%).
According to a Deloitte survey, the majority of financial services AI adopters (86%) consider AI crucial to their organization’s success in the upcoming two years.
Among the largest European and American banks, the top three organizations with the highest AI readiness index are Capital One, JPMorgan Chase, and Royal Bank of Canada. The runners-up are TD Bank, ING, Citi, HSBC, UBS, BNP Paribas, and BBVA.
As for the current use of AI in banking and finance, the top use cases worldwide are data analytics, data processing, natural language processing, and large language models.
Increased operational efficiencies are the primary benefit financial organizations reap from AI implementation. It’s followed by competitive advantages and improved customer experiences.
How has Al improved your business operations? (excluding China)
Created operational efficiencies 43%
Created competitive advantage 42%
Improved customer experience 27%
Yielded more accurate models 27%
Opened new business opportunities 23%
Reduced the total cost of ownership 14%
AI Investments in Finance Expected to Rise
The banking sector is the leader in investing in AI systems across industries. Worldwide, banking organizations invested $20.6 billion into AI capabilities in 2023 alone, representing 13.37% of all global AI investments ($154 billion).
Financial services organizations overall are also expected to increase their AI spending significantly at a CAGR of 29% between 2023 to 2027, from $35 billion to $97 billion.
The top use cases financial services organizations are investing in are:
- Risk management (36%)
- Portfolio optimization (29%)
- Fraud detection (transactions/payments) (28%)
- Algorithmic trading (27%)
- Document management (26%)
- Customer experience (26%)
The banking sector’s investments in generative AI in particular are expected to skyrocket from $3.86 billion in 2023 to $85 billion in 2030, with a CAGR of 55.55%. And that’s understandable: those investments are expected to generate an extra $200 billion to $340 billion, or 2.8 to 4.7%, in annual revenue worldwide via a productivity boost.
51% of financial services organizations surveyed by NVIDIA plan to invest in identifying additional AI applications in the future, making it the number one investment target area. The runners-up are AI workflow, production cycle optimization, and infrastructure spending.
How do you plan to invest in Al technologies in the future? (excluding China)
2023 2022
Identify additional Al use cases 51% 40%
Optimize Al workflow/production cycle 37% 37%
Spend more on infrastructure 26% 26%
Engage third-party partners to accelerate Al adoption 26% 19%
Provide Al training to staff 21% 28%
Hire more Al experts 20% 28%
Key Market Players
According to Mordor Intelligence, the market remains somewhat fragmented, with high competition and no clear dominant players that would consolidate it.
Key players in the market include (in no particular order):
- IBM Corporation. The company is recognized as a Leader in the 2023 Gartner Magic Quadrant for Finance and Accounting BPO. It offers comprehensive AI consulting and implementation services for financial services organizations. Its GenAI solution, watsonx, allows companies to launch their custom models. IBM’s clients include NatWest, Capital Bank of Jordan, and ABN AMRO Bank.
- Intel Corporation. Intel offers AI software tools and optimizations, Intel Developer Cloud, and hardware for AI developers. The company recently unveiled its AI accelerator chip in an attempt to challenge NVIDIA’s capabilities in the domain.
- ComplyAdvantage. The company offers an AI-driven fraud and anti-money laundering risk detection solution to financial services organizations. Its clients include Munich RE, Allianz, OakNorth Bank, and Santander.
- Amazon Web Services (AWS). AWS offers a wide variety of development tools for AI solutions, from computer vision (Rekognition) and chatbots (Lex) to fraud detection (Fraud Detector). Its clients include MasterCard, Resolution Life, and NatWest Group.
Ethical AI Use: 5 Considerations to Keep in Mind
While there are many benefits of AI in finance, the ethics of using technology to automate processes and make decisions is a topic bound to dominate the discussion this year. Regulators, consumers, and financial services organizations alike have to pose the question: Can you trust the AI system?
For now, consumers are undecided whether GenAI finance chatbots are trustworthy: 56% of finance customers are neutral when it comes to trusting them, and only 21% fully trust them.
At the same time, the ethics of AI use seem to mean different things to different organizations. The 2024 AI Index report revealed the discrepancies in self-reported responsible AI benchmarks for popular foundation models:
That said, IBM’s responsible AI framework can serve as a solid basis for ensuring you can trust the AI system’s output.
Here are the five pillars of trust that you can use to ensure ethical AI use in your financial organization: explainability, fairness, robustness, transparency, and privacy.
Explainability
Certain AI systems come with the black-box problem. It means not even developers can fully understand how the model obtained a specific output in a specific case. In finance and beyond, this poses the problem of algorithmic bias.
As AI systems learn to produce output by detecting patterns in historical data, they may inadvertently automate discriminatory practices in credit decisions, customer service, and investment strategies. This isn’t a new issue: the U.S. Treasury Department warned about the “potential for fair lending violations” in 2016.
Explainable AI is meant to solve the black-box problem by requiring the system to provide the reasoning behind its output.
Ensuring AI explainability means:
- Verifying the system’s prediction accuracy by comparing the output to the results in the training set
- Enabling result traceability to enable users to track the system’s processes
- Educating frontline users on how AI systems reach their conclusions to enable better human oversight
Fairness
To prevent algorithmic bias from informing high-stakes decisions that impact consumers, an AI system has to be designed with fairness in mind. This means:
- Using diverse and representative training data sets to avoid underrepresentation or bias
- Checking the data for biases and correcting them before training
- Tracking fairness metrics to monitor disparities across demographic groups
- Training the AI model to self-correct behavior based on predefined fairness criteria
- Employing re-sampling, adversarial training, re-weighting, and other anti-bias techniques in training
- Creating an ethical AI review board or committee to oversee the ethical implications and risks of AI use
- Keeping a “human in the loop” to enable human oversight and intervention
Robustness
AI models are increasingly trained on sensitive data and proprietary information, and this makes them a target for malicious actors. To protect AI systems from attacks, developers should train them to respond to anomalous input and malicious attacks without revealing any sensitive data. AI models should also be stress-tested to identify and patch up any potential vulnerabilities.
Transparency
Users have to be informed about the involvement of AI in specific processes, from the specific purpose the AI system serves to its strengths and limitations. This goes hand in hand with educating users on how AI functions and how to spot potentially inaccurate or misleading output.
Privacy & Data Security
In finance, AI models tend to be trained on a wide variety of personal data. However, financial services customers value transparency about how their data is used. Furthermore, 78% of consumers are ready to switch providers if they believe their data is mishandled.
To ensure your use of AI in finance doesn’t alienate your customers:
- Train AI models on as little personal data as possible and anonymize data whenever avoiding personal data use isn’t possible
- Consider using synthetic data sets for training AI
- Clearly communicate to customers when and how their data is used and enable opting out
- Properly secure the training data in accordance with legal requirements (e.g., GDPR)
4 Challenges of Adopting AI and ML in Finance
Using AI in finance comes with four key challenges, according to NVIDIA’s 2024 report: data issues, talent shortages, budget constraints, and insufficient data.
What are the biggest challenges in achieving your company's Al goals? (excluding China)
Data issues: privacy, sovereignty, and disparate locations 38%
Recruiting and retaining Al experts and data scientists 32%
Lack of budget 28%
Sufficient data sizes for model training and accuracy 27%
Data Issues
This is the number one struggle for financial organizations looking to adopt AI. It surpassed the talent shortage in 2024, which was the greatest concern the year before.
Data issues include:
- Maintaining privacy
- Implementing a robust data management and governance strategy
- Aggregating data from multiple jurisdictions with varying legislation
Talent Shortage
AI experts and data scientists are in short supply, leading to difficulties in recruitment and retention for financial organizations looking to ramp up their AI capabilities in-house. Another survey conducted by EY corroborated that talent shortages are a challenge for 44% of respondents looking to adopt AI in financial services.
Budget Constraints
A lack of sufficient investment in AI adoption can hinder any initiative. This can be due to many reasons, among which is that a lack of buy-in from leadership may be key. According to EY’s survey, 36% of industry leaders cited a lack of clear commitment as a top barrier to adoption.
Insufficient Data
AI models have to be trained on vast amounts of data, but obtaining it is a struggle for around a quarter of surveyed financial organizations.
At the same time, data volumes aren’t the only obstacle here: data quality, arguably, matters even more. Poor quality is one of the four key barriers to AI adoption for three-quarters of FSI executives, according to Deloitte.
4 AI Opportunities to Seize in the Finance Industry
Cutting-edge implementation of AI and machine learning in finance can come in the form of synthetic data and report generation, enhanced risk management, and real-time portfolio optimization.
Report Generation & Data Extraction
Report generation is the number one GenAI use case explored by financial services organizations. LLMs can process and summarize information in a given format in a matter of seconds, increasing employee productivity as a result.
Common documents that can be automatically generated include risk assessment, fraud detection, and investment performance reports.
NLP and OCR, in turn, can also automate data entry and analysis. Information from invoices, reports, contracts, and statements can be automatically extracted and structured, and an LLM system can then be used to summarize the information.
Synthetic Data Generation
According to NVIDIA’s survey, 33% of financial services organizations are exploring the use of GenAI for synthetic data generation.
This application can be a solution to a pressing data issue: proprietary data necessary for training AI and ML models may be locked in various jurisdictions. In such a case, generative AI can be used to create synthetic datasets that replicate the said locked-in data.
Enhanced Risk Management
Risk management is the leading AI use case financial services organizations are investing in today. It includes advanced payment and transaction fraud detection and continuous AML and KYC compliance monitoring, among others.
In KYC compliance, advances in deep learning models (e.g., graph neural networks) make pattern detection across entities more efficient.
Real-Time Portfolio Optimization
While AI has been used in portfolio management for a while now, its ability to derive insights from real-time data feeds got financial organizations interested. AI-powered portfolio optimization is the second leading investment area, with 29% of surveyed organizations investing in it.
However, AI isn’t seen as a means of completely automating the portfolio management job. It’s meant to be a copilot for managers, instead.
How Collaboration Impacts the Financial Services Industry
Bank and credit union executives traditionally view big tech and fintech companies as competitive threats.
This trend is even more pronounced in 2024. Cornerstone Advisors revealed a sharp year-on-year increase in the share of executives considering big tech and big fintech significant threats in the coming decade.
However, that is not to say collaboration has no place in the finance industry. The same Cornerstone Advisors survey revealed that 70% of bank and 80% of credit union executives view fintech partnerships as a moderate or significant driver for growth.
When it comes to AI, U.S. Bank is one of the most recent examples of collaboration between banks and fintech startups. The Minneapolis-based lender partnered with Pagaya Technologies to introduce AI into its credit decisions for personal loan applications. Wells Fargo, in turn, entered a partnership with TradeSun AI to streamline trade finance and compliance operations.
Traditional financial institutions also increasingly seek the expertise of big tech companies to get ahead on AI adoption. Notable players in AI and ML in finance include NVIDIA (e.g., multi-year partnership with Deutsche Bank), Microsoft (e.g., AXA’s SecureGPT), Google, and AWS.
Although collaboration within the private sector is most prominent, certain players team up with academia, as well. For example, PNC Bank partnered up with Carnegie Mellon University to establish the PNC Center for Financial Services Innovation. The Center focuses on researching and advancing interpretable ML marketing models for banking.
4 Future Trends to Keep an Eye On
The future of AI in finance is likely to be shaped by explainable AI, advances in hyper-personalization, decentralized finance, and the increasing use of AI and machine learning in finance risk management and compliance.
Explainable AI
The explainable AI market size is expected to almost quadruple to over $24 billion between 2023 and 2030, according to NMSC.
84% of organizations surveyed by NVIDIA are already using tools to ensure their AI remains trustworthy and explainable. The same share of organizations is planning or implementing a governance framework for their use of machine learning and AI in finance. Their goal is to ensure both regulatory compliance and alignment with business goals.
Some regulators are also proactively pushing for explainable AI use in finance. For example, certain policymakers in the European Union and Asia have already adopted regulations to enable consumers to request an explanation for any AI-generated decision.
While explainable AI is likely to be the future of AI, it comes with certain tradeoffs. The most notable one is the model accuracy: the more interpretable it is, the lower its accuracy tends to be. Furthermore, explainable AI computations require more resources and, therefore, are slower in producing output.
AI-Powered Hyper-Personalization
According to Salesforce’s survey, 61% of banking customers want personalized experiences. The rates are even higher among insurance (66%) and wealth management (73%) customers.
As fintech companies exert increasing competitive pressure, incumbent organizations have to step up their game in personalization efforts. However, two out of three banks remain incapable of assessing the customer’s situation beyond a single point in time. This results in increased costs, lower business agility, and poor customer experience.
To meet the demand for personalization, financial services organizations have to serve each customer as if they’re a “segment of one,” as Deloitte put it. Such hyper-personalization is impossible without AI systems that analyze and predict behavior, execute micro-segmentation, and analyze customer journey metrics—all in real time.
Decentralized Finance (DeFi)
Although the DeFi market has declined since its peak in 2021, in terms of total value locked and user count, the market is still valued at $48.65 billion. In this sector, AI can resolve two inherent challenges of decentralized financial services: fraud detection and risk assessment.
AI can, for example, analyze blockchain transaction data to assess potential borrowers’ risk profiles in DeFi lending. As for fraud detection, the technology’s application is similar to the AI used in finance overall. The system can monitor transactions in real time to detect anomalies or suspicious behavior.
Furthermore, AI is already being combined with smart contracts in parametric insurance. In this combination, AI can analyze data feeds (e.g., weather data) to identify when an insured event occurs (e.g., a natural disaster in the policyholder’s area). The event triggers an automatic payout of a predefined amount.
For example, Chainlink allows the design of smart contracts for parametric weather, crop, flight, and travel insurance.
AI in Risk Management & Compliance
Risk and compliance is already the second business function with the highest AI adoption rates. 45% of organizations leverage AI to streamline certain tasks. Risk management is also the top AI investment in the industry.
Generative AI, however, represents a new level of productivity and efficiency gains in risk and compliance. Albeit the assessed impact of generative AI on risk and legal in banking and insurance is moderate, the technology is poised to streamline multiple tasks, such as:
- Creating reports
- Summarizing regulations
- Responding to questions about applicable regulations and policies
- Identifying policy or procedure misalignment
For example, Citigroup reportedly leveraged generative AI to create a summary with key takeaways of new capital rules on the U.S. banking sector, which totaled 1,089 pages. With its help, the organization’s risk and compliance team could assess the impact of new regulations faster.
How Regional Differences Impact AI Use in Finance
Each region represents a unique set of regulatory requirements, adoption trends, and market dynamics, making a one-size-fits-all approach to AI and ML in finance impractical.
The disparities are most noticeable in consumers’ knowledge of and attitudes toward AI:
- Over two-thirds of Indonesians and Malaysians know which products and services use AI, as opposed to 35% in Belgium, New Zealand, and the U.S.
- Excitement about AI tools is also the highest in the developing regions and lowest in Europe and North America. Trust toward AI ranges from 72% in Thailand to 32% in France, Japan, and the U.S.
Here’s how three different regions are embracing AI in finance.
United States
The U.S. is leading in the number of notable machine learning models and hosts over a third of the world’s AI companies. With an estimated 15,000 AI companies in the U.S. market, the country is also the leader in private investment in AI, outpacing the closest runner-up sixfold.
Regulatory Overview
The use of AI in the U.S. is coming under increasing regulatory pressure: the number of regulations increased between 2022 and 2023. That said, the use of AI in finance remains largely governed by a patchwork of federal agencies’ requirements and recommendations, along with disparate state regulations.
President Biden’s executive order, while aiming to push for stricter regulations on the federal level, is unlikely to lead to a federal bill passed in an election year. More guidelines on AI use in finance will likely come from the Consumer Financial Protection Bureau in 2024.
Adoption Trends
In 2023, a third of the U.S. population, around 90 million people, was already using AI tools. The number of AI users is expected to rise to 241 million by 2030.
However, U.S. consumers are becoming increasingly concerned about AI in their daily lives, with the rate of those more concerned than excited rising from 37% in 2021 to 52% in 2023.
Ipsos found that only 37% of U.S. respondents believe AI benefits outweigh its drawbacks. Furthermore, only 36% of U.S. consumers trust companies that use AI as much as those that don’t.
Around a third of U.S. organizations have already adopted AI, with the top use cases being data analysis, cybersecurity, design tasks, and financial analysis. According to another study, 19% of U.S. businesses don’t use or plan to use AI, 33% have deployed AI systems, and 38% are exploring AI use.
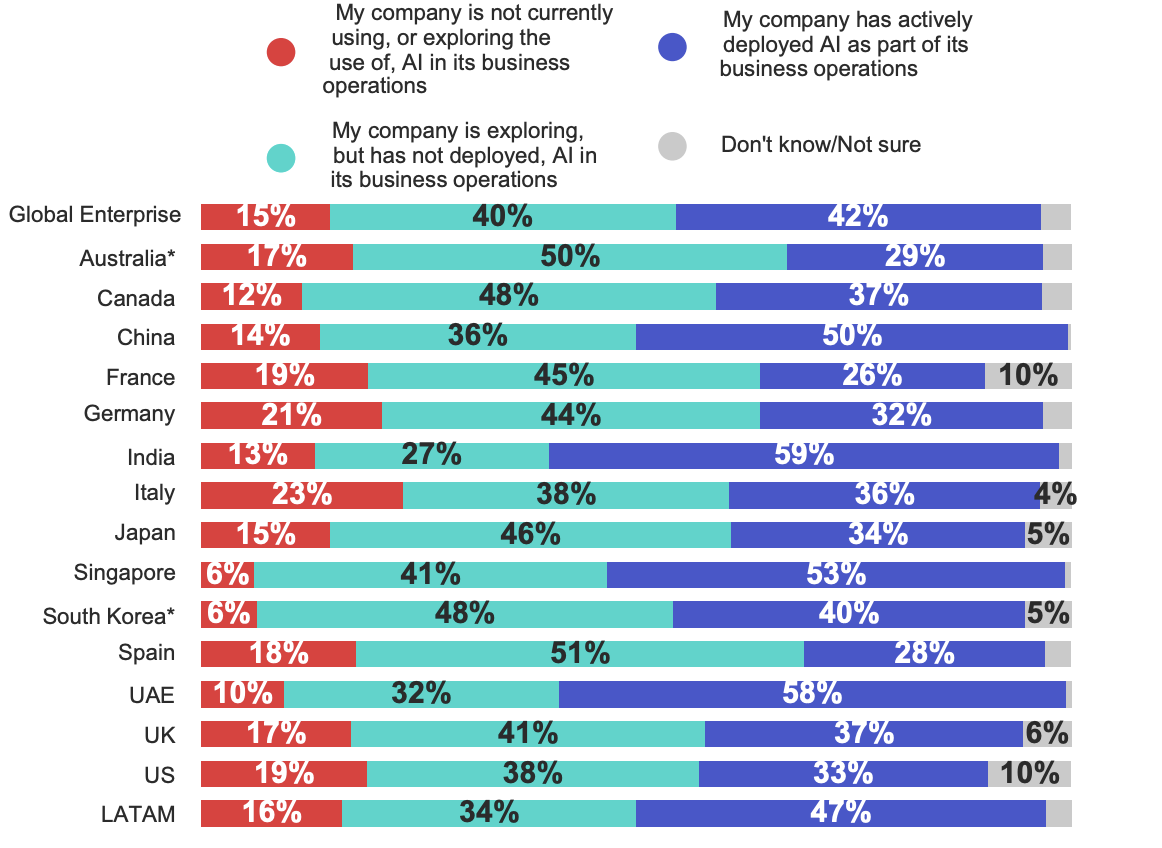
IBM Global AI Adoption Index Report 2023. company adopted or explored using Artificial Intelligence (AI) as part of its business operations and digital transformation
Market Dynamics
The U.S. AI market is expected to grow from around $90 billion in 2023 to over $200 billion in 2030. However, it’s worth noting that the market has been somewhat volatile between 2020 and 2023, with both sharp increases and slumps.
The United States is also a leader in private investment into AI on the global stage, with it reaching $67.2 billion in 2023. This puts the U.S. far ahead of the EU and the UK ($11 billion) and China ($7.76 billion).
European Union
While the European Union arguably leads the way in regulating AI, the region is considerably behind the U.S. in the number of AI companies present (estimated at 6,000). While it is showing substantial AI adoption among consumers, adoption rates are lagging behind those of the U.S.
Regulatory Overview
The most pressing regulation financial services organizations must address is the AI Act. Although the AI Act is still awaiting a final lawyer-linguist check and a formal endorsement from the Council, it’s likely to be fully adopted before the end of the legislature.
Financial services organizations will have 36 months to ensure compliance with high-risk system provisions after the AI Act enters into force.
In addition to the AI Act, AI adopters in the EU have to pay close attention to the DORA regulations, as well as the GDPR.
Adoption Trends
EU’s key AI markets are lagging behind in adoption rates, with Spain and France showing some of the lowest rates of AI adoption in large organizations (28% and 26%, respectively). Another study found that only over a third of European businesses are adopting generative AI in their operations.
On the consumer level, around 80 million EU residents use AI tools as of 2023, despite being home to a larger population than the U.S. (444.8 million vs 336 million). However, the number of AI users is expected to triple to almost 250 million by 2030, potentially outpacing the United States.
Most EU consumers want governments to put guardrails on AI adoption. One survey showed that 68% of Europeans want regulations to protect jobs from generative AI-powered automation, with an increase from 58% in 2022.
Another study revealed discrepancies in attitudes toward AI across EU member states. Romania (61%) and Italy (53%) have the highest rate of respondents believing AI’s benefits outweigh drawbacks. France (37%) and Sweden (39%), however, ranked closer to the bottom. A similar picture presents itself when it comes to trust in AI among consumers.
Market Dynamics
The AI spending in Europe was estimated to reach $34.2 billion in 2023, with $5.37 billion in the banking sector. That makes the industry responsible for the most considerable value of AI investment in the region.
The EU authorities are actively investing in AI to promote its development and adoption. For instance, its Next Generation EU initiative recently committed €4.4 billion to AI initiatives, with Italy, Spain, and Germany being the top three investment recipients.
China
China is the runner-up in AI capacities, ranking second in the number of notable ML models and first in the number of AI patents. While it’s one of the key AI adopters in the world, the Chinese government’s overreaching regulatory control may be stifling innovation in the field.
Regulatory Overview
China was the first country to implement binding regulations on general AI use in 2021 and 2022. The regulations first focused on recommendation systems and watermarking AI-generated images and videos. The former impacted the finance industry as much as news outlets, search engines, and social media platforms.
However, the expanding censorship regulations are thought to stifle innovation in China, leading to shutdowns and layoffs and putting China behind in generative AI progress.
China is also expected to follow the EU’s steps and adopt a general AI law in 2024. This comprehensive law, while on the agenda, is currently in the drafting stage. The advisory draft published in 2023 contains a negative list of areas companies have to avoid engaging in unless they have the government’s approval.
Adoption Trends
Half of large organizations are already using AI in operations, and 85% of them are planning to accelerate AI rollout, according to IBM’s 2023 Global AI Adoption Index.
Another study showed over 80% of China-based enterprises are exploring AI implementation or have already deployed the technology, making it the country with some of the world’s highest AI adoption rates in the workplace.
Market Dynamics
Finance is one of the three industries with the highest AI adoption rates in China. Along with retail and high tech, it makes for a third of the country’s AI market, with the financial services AI market sector valued at 38 billion yuan. The key applications of AI and machine learning in finance in China include insurtech solutions and robo-advisors.
However, while the country has long been a leader in the development of AI, its generative AI capabilities are lagging behind those of the United States by at least a year. The latter’s restrictions on exporting advanced chips to China, necessary for training cutting-edge AI models, are also hindering the country’s ability to innovate.
On the other hand, the country is leading in facial recognition technology: the five most accurate facial recognition models are developed by Chinese firms.
5 Technologies to Shape the Future of AI in Finance
AI encompasses a variety of technologies and some of its subsets are especially significant for its future, including machine learning and natural language processing. Also, AI is often implemented together with robotic process automation. Additionally, its evolution is influenced by other technologies such as blockchain and quantum computing.
Machine Learning (ML)
Machine learning is a subfield of artificial intelligence. ML models leverage data and algorithms to simulate how humans learn, enabling them to identify patterns and make predictions.
Common ML algorithms include neural networks, linear and logistic regression, clustering, decision trees, and random forests.
Machine learning is integral to making computer vision, natural language processing, and speech recognition work, among others. However, in finance, its main application lies in data analytics and processing. These two capabilities are the most common AI workloads used by financial organizations today.
According to Cornerstone Advisors’ report, the adoption of ML among banks rose from 2% in 2019 to 16% in 2024. Results are similar for credit unions, where the adoption rates rose from 2% in 2018 to 21% in 2024.
Natural Language Processing (NLP)
Natural language processing uses ML algorithms and computational linguistics to enable machines to recognize and process user input in the form of natural text or speech. NLP enables software tools to translate and summarize text, recognize spoken commands, and assess the sentiment of a written or spoken input.
Natural language processing is the third most common AI/ML capability in finance, used by 47% of financial services organizations. It’s essential in developing virtual agents and chatbots, as well as sentiment analysis tools and voice-user interfaces. NLP is also used to process user input in generative AI tools.
Robotic Process Automation (RPA)
Robotic process automation (RPA) tools allow users without coding experience to create robots that automate repetitive tasks in a given workflow.
RPA can be combined with AI, but it’s not always the case. On its own, bots created with RPA tools follow rule-based algorithms. They can repeat the user’s steps in copying and pasting data or filling forms, for example. With AI, however, RPA agents can take on more complex tasks, such as understanding unstructured data and organizing it into structured templates.
RPA combined with AI is already in use at 27% of banks and 32% of credit unions, according to Cornerstone Advisors. The technology has come a long way compared to 2018, when only 4% of banks and 2% of credit unions deployed RPA tools. Another 16% of banks and 20% of credit unions are planning to invest in or implement RPA in 2024.
Table G: Banks' Al Plans for 2024
Have already invested in or deployed Planning to invest and/or implement in 2024 Have discussed at the board or executive team level Not on our radar
Robotic process automation 27% 16% 24% 34%
Chatbots 20% 13% 42% 24%
Machine learning 16% 17% 37% 30%
Generative Al 6% 14% 53% 27%
Source: Cornerstone Advisors survey of 359 community-based financial institution executives, Q4 2023 and Q1 2024
Blockchain
A blockchain is a distributed ledger that stores a sequence of records (blocks) across a peer-to-peer network. As records are stored on multiple machines, blockchain offers a transparent way to do it. The ledger is also designed to be immutable: transactions can’t be reversed as records can’t be removed from the chain.
Cryptocurrencies, smart contracts, and non-fungible tokens all rely on blockchain technology. Smart contracts, however, are the most promising application of blockchain in finance. They automate the execution of the contract (e.g., payment) when certain conditions are met (e.g., delivery takes place).
AI can find its application in smart contract oracles which pull data from off-chain sources. The technology can analyze the off-chain data to assess its accuracy and reliability and weed out manipulated data to prevent fraud.
Quantum Computing
Quantum computers represent a different approach to computing, which relies on quantum mechanical phenomena. Instead of using bits that can store either ones or zeros, quantum computers’ qubits can store both. As a result, quantum computers can execute complex calculations times faster compared to traditional computers.
Quantum technology is seeing record investment, with quantum startups securing $2.35 billion in 2022 alone. While McKinsey estimates that a quantum computer won’t be viable at scale by 2040, governments around the world have pledged to invest $34 billion into researching the technology.
As AI models are designed to run complex computations, quantum computers would allow for the creation of more advanced models that process more variables from more data points. Quantum computers would also significantly speed up training AI models.
How AI is Transforming Financial Services: Expert Opinions
In June, S-PRO hosted the webinar "How AI is Transforming Financial Services". This discussion brought together 2 industry leaders to talk about the impact of AI on the finance industry, and the importance of mastering concepts like machine learning, deep learning, and generative AI. Citing studies by McKinsey and Capgemini, this webinar illustrated AI's potential to increase operational profits and streamline processes like documentation and call center automation. However, challenges such as legacy systems, regulatory complexities, and data fragmentation were also discussed, along with risks like bias and privacy concerns.
The panel speakers Ephraim Frei and Matthias Zwingli shared their unique experiences.
Ephraim Frei, the Head of Corporate Clients at S-PRO. With several years in tech and corporate finance, Ephraim has been at the forefront of integrating smart solutions into banking. His journey into AI and ML began around 2017 while studying at university with assignments on deep learning. This initial exposure led him to a fintech firm, where he worked on AI applications such as OCR and text categorization. In 2021, amid the pandemic, Ephraim joined S-PRO, a leader in AI, blockchain, and custom software development. The S-PRO team reinforced his belief in AI's potential to reshape the finance industry.
Matthias Zwingli, CEO and Founder of Connect AI, has a rich background in innovation and technology, with over a decade of experience. His company offers tailored AI applications for businesses across various industries, reflecting his deep engagement with AI and machine learning. Matthias's journey into AI was sparked by his fascination with the "black box" nature of AI, which he sought to demystify despite his primarily business-oriented background. This curiosity led him to dive into the technical side of ML, including a bootcamp in Python coding. This practical involvement has not only broadened his understanding but also driven him to apply these technologies, demonstrating AI's impact.
Why is the Potential in the Financial Services Industry So Great?
The potential of AI in finance is immense, as illustrated by the estimated value creation in various sectors of the finance industry. Generative AI alone is expected to add between $200 billion and $340 billion to banks, highlighting the transformative impact of AI apps across different segments. In corporate banking, AI and machine learning could generate up to $321 billion, offering enhanced decision-making capabilities and streamlined operations. Retail banking and risk management also stand to gain significantly, with potential contributions of $306 billion and $385 billion, respectively, through improved customer insights and robust risk assessment models.
The adoption of advanced AI and ML technologies can turn traditional practices in banking upside down, from fraud detection to personalized financial advice. In areas like asset management and wealth management, AI can optimize investment strategies and deliver personalized services. Moreover, the integration of AI in software engineering and IT further drives innovation, making financial services more efficient and secure. This broad scope of potential benefits underscores why the financial services industry is a fertile ground for AI-driven advancements.
Source: McKinsey 2023
The Challenges of Implementing AI in the Finance Industry
“How do you measure the success of AI? Usually, it's accuracy, but if you take accuracy, then you will never reach the full potential. Because the human mind also makes mistakes. So there needs to be a room for more points to measure and use cases which are not that risky at the beginning,” says Ephraim Frei.
Despite the transformative potential of AI, the finance industry still faces challenges in integrating AI applications. Legacy code bases and system incompatibilities hinder the adoption of modern machine learning solutions, as outdated infrastructures are costly and technically difficult to upgrade. Additionally, fragmented data across various systems complicates the consolidation and data integrity.
Regulatory complexity makes implementing AI in finance challenging, because meeting strict standards is both time-consuming and costly. The shortage of skilled professionals also complicates things, making it difficult to develop and manage advanced AI systems. Additionally, financial institutions often show risk aversion and are hesitant to invest in AI due to the high costs and uncertain returns.
Ensuring customer trust is also crucial; AI systems must be transparent and fair, especially in sensitive areas like credit scoring. Identifying suitable use cases for AI and balancing budget allocation between innovation and operational needs are additional hurdles. The shift towards cloud computing presents challenges related to data security and compliance, while eliminating data silos and addressing centralization complexities require significant organizational changes and cooperation.
The Risks of AI in Finance
However, there are still many things that need to be done to achieve the goal of the finance industry managing risks related to the implementation of AI applications. Bias in AI can result in discrimination, for example, in credit rating and loaning systems, this can cause questions on fairness and the need for regulations. Privacy risk is also important because the AI and machine learning algorithms work with large amounts and types of data, which makes data leakage and unauthorized access to personal data possible. Matthias Zwingli highlights in the webinar:
“You have to be very aware of which data you're going to use, what you want to build on, and how crucial the outcome is.”
Recommendations that are based on hallucinations, where the information provided by AI is wrong, are a risk for banking and corporate finance as wrong decisions are made and money lost. However, there are dangers of using AI for malicious purposes like phishing, or for managing the stock market, which requires good protection from fraud and proper regulation of the use of AI in finance.
6 Major AI Trends Shaping 2024
“What's going to be super interesting in 2024 is Llama giving an open source. They're going to massively improve performance. I think it's kind of crazy. I don't know if we've had it in the history of humankind, but we have state-of-the-art, best-in-class AI models just democratized and accessible for everyone,” says Matthias.
In 2024, 6 major AI trends will significantly impact the finance industry. First, AI systems will become increasingly multi-modal, enabling them to see, hear, talk, and interact in more human-like ways, enhancing AI applications in customer service and fraud detection. Second, these systems will better interact and connect, seamlessly reading and writing across diverse data sources, improving decision-making in banking and corporate finance.
Third, advancements will make AI control easier, ensuring more consistent outputs, which is crucial for maintaining regulatory compliance and operational stability. Fourth, AI will become more democratized and accessible, with low-code platforms allowing non-experts to develop and deploy solutions, broadening the use of machine learning across financial services.
Fifth, the rise of AI platforms and marketplaces will allow for the easy publication and sharing of AI models, facilitating innovation and collaboration. Lastly, declining costs and cheaper APIs will make advanced AI technologies more affordable, allowing smaller financial institutions to leverage AI and ML.
Summary
The applications of AI in finance are poised to make an impact on every aspect of financial services operations. The technology is already creating operational efficiencies and competitive advantage, improving customer experience, and opening business opportunities across risk and compliance, operations, and marketing and sales.
To turn AI into the potent productivity multiplier that it can be, however, financial services organizations have to navigate multiple challenges. The most pressing ones are the rapidly evolving regulatory landscape, data and privacy challenges, and consumers’ concerns over AI use in certain markets.
Addressing these challenges requires careful consideration of the ethical implications of AI use – and strong governance and oversight over AI tools.